Initial AI journey and Proof of Concept
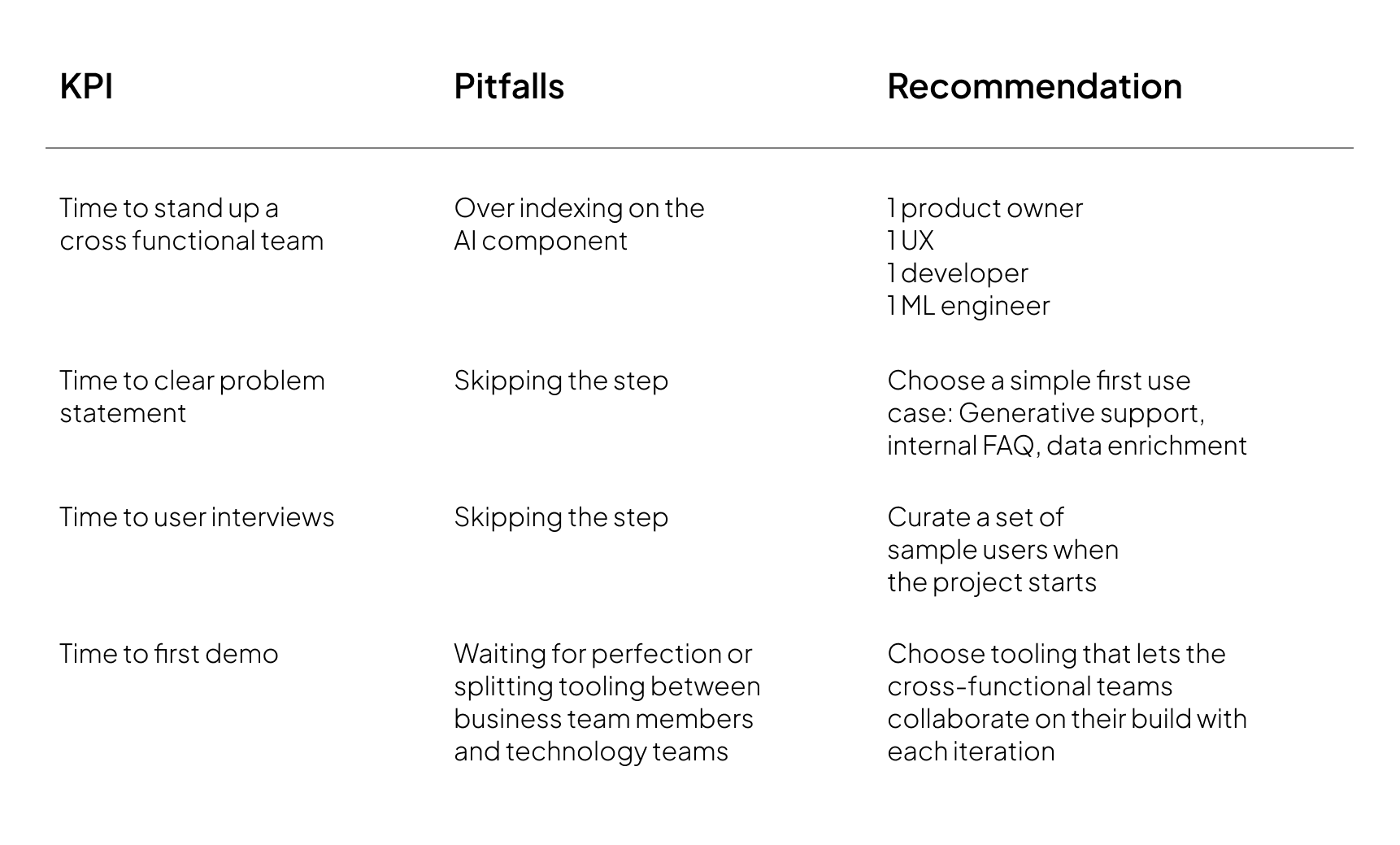
Your initial AI project should be about clearing the list of unknowns. It’s essential to iterate on ideas and demos to create a first implementation. This work falls into three buckets - team coordination, delivery speed and user iteration speed.
As a leader, your core objective is to unblock teams and provide a space to iterate and improve AI products. Each of these objectives should come with their own key performance indicators to track and measure time until delivery.
Leaders need to structure a team that’s set up for success. Many generative AI projects have been staffed exclusively within a ML or IT team, ignoring cross-functional needs to build strong customer experiences and corresponding business cases. This team should be capable of deep diving into a problem area and creating clarity in a fast paced space. Although not a strict list, successful POCs typically have inputs from a product owner, UX, developer and ML engineer.
Clearly defining a problem statement for your POC will help teams deliver with focus and avoid getting lost in AI distractions. In many circles, AI has become a solution looking for a problem, which is great for initial momentum, yet returns in fury and creates lasting tech debt in years 2-3 of a 5 year AI strategy.
User interviews often forgotten in the push to build. Curating a beta group is a highly valuable way to measure product improvements, whether these users are internal or external. These iterations should be quick, with notes shared across the entire team to understand key concerns and positive outcomes. Often times this step is skipped as engineering teams focus on the build and only at the last moment deliver a sharable experience.
The most important item is getting a cohesive demo prepared, including a clear value proposition and budget for taking the project to the next step. The initial proof of concept is designed to de-risk a project, but success is measured by projects’ progress. Without progress many teams get stuck in proof of concept purgatory, where these early use cases keep getting built with no clear next step for production or past first launch.
Presenting a cohesive narrative for moving the POC to production becomes essential.
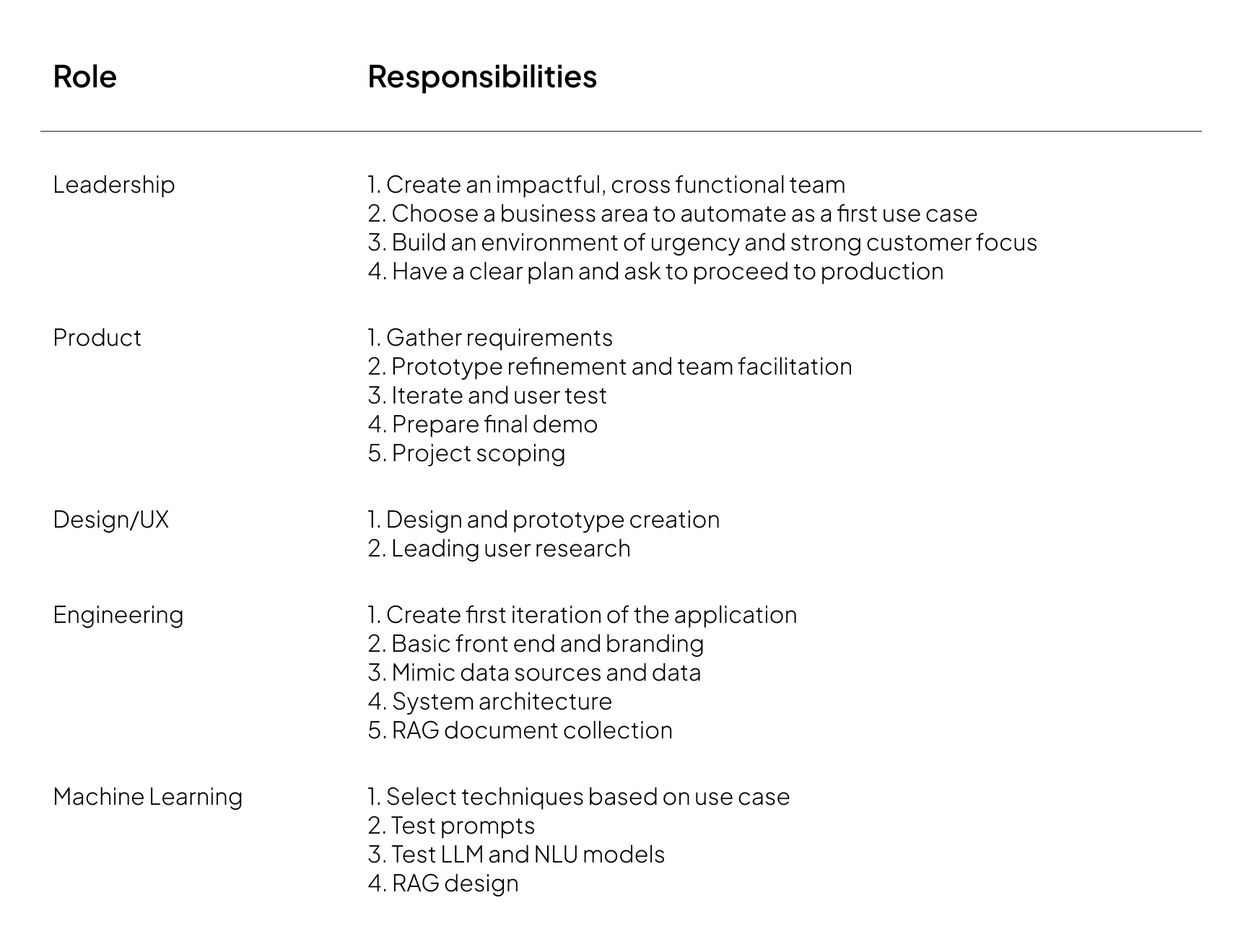
Deploying to Production
KPIs:
- Time to connect to production data
- Time to move between product environments
- Time to build an evaluation suite
- Time to finalize UI
- Time to complete security and risk assessments
- Time to launch feature
As clearer use cases are defined, more teams become involved in the process. With more teams, clear communication becomes essential for progress and alignment. To navigate this complexity, it’s important that feature and product launches are well managed and the tooling reflects this collaborative, scaled effort.
Connecting to production data is essential at this step. In a proof of concept environment we often focus on mimicking production data or using a subset since integrating with existing systems can be challenging. When moving into production, this should be a key task for the engineering team to connect and test the POC with production data. Depending on the organization, this data may sit in a higher environment, requiring the team to deploy to an user acceptance testing environment (UAT).
Next, teams should focus on speed to move the features through each stage of the product environment. Typically there are 2-4 environments depending on the stage of the company, but similar to software projects, fast progress times through environments leads to faster iteration cycles.
On the engineering side of the house, the team should be measured on creating strong testing metrics and brand aligned front-ends. Testing is particularly challenging since generative AI models are non-deterministic, requiring tests to validate how users can interact with the models. This work will typically be done in conjunction by a user testing, ML and engineering team to define and deliver on thorough and relevant tests. When considering the front end, generative AI applications should feel like a natural extension of an existing application, rather than being bolted on as an afterthought. This is where design, UX and front end teams need to collaborate to create the integration. To speed up the delivery process, using pre-existing open source or hosted front end frameworks can be strongly beneficial.

Beyond product development often lies a negotiation with the security, compliance, and risk teams. Generative AI security and risk policies are still being defined or iterated on within many organizations, and navigating these policies can slow down projects. Ideally these discussions are started in the POC phase to fully align teams early. We discussed some of these approaches in a prior piece.
Launching the feature live is the final milestone. Once the first application goes into production, there will likely be more projects starting, so the KPIs can apply across new projects across the organization. It’s important that leaders have set a clear product roadmap and teaming structure so that each new use case and iteration is not additional technical debt, rather an additive product lifecycle that becomes more efficient with each new launch.
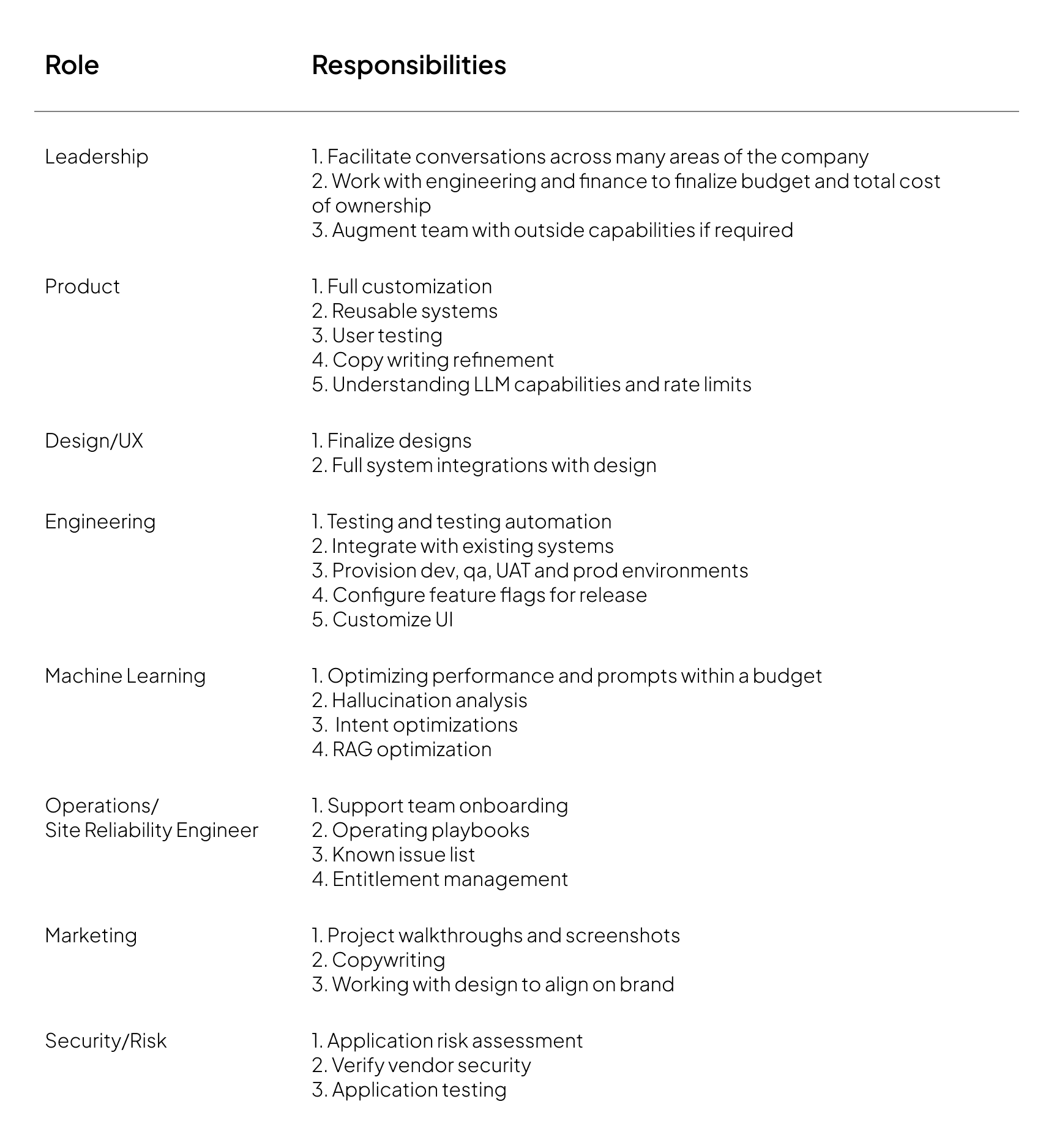
Iterate and Scale
KPIs:
- Time to make simple updates
- Time to launch subsequent version
- Time for next team to launch product
- Cost per additional project
- Total cost of ownership
After deploying at least one use case to production, the challenge for an organization is to scale their generative AI applications across teams in repeatable and financially sustainable way. Competitive advantage is created when teams can build with an innovation mindset and not be stuck in maintenance mode. The KPIs for this phase focus on this agility and cost.
It’s first important to measure the ability to quickly update your AI applications. Simple changes like minor prompt updates, copywriting, or API version bumps should not be complex processes. Similar to rapid software release, making small changes to generative AI updates, especially after initial release, builds trust with customers and address minor oversights.
What about larger iterations? Models, data and product requirements continue to evolve quickly, so being able to update features and capabilities will future proof your generative AI products from becoming legacy product lines (e.g. an AI chatbot on your homepage that was launched as an initial use case, yet quickly becomes ignored and outdated without a proper team, workflow, and iteration cycle).

Similarly, as adoption increases across an organization through new use cases, teams should be able to onboard more quickly after the initial use case. The total cost of ownership for each new team and use case should become more efficient as the level of innovation and automation across an organization ramps exponentially. At the CIO level, this should be an essential metric when implementing and scaling your AI strategy.
The final two KPIs in this section focus on cost, both the marginal cost per application and the total cost across a strategy. These two metrics help organizations plan future projects and measure the ROI across their generative AI initiatives. They also help scope investments to be leaner and more deeply integrated within a technology initiative, rather than a separate line item with a large commitment.

Conclusion
As teams build their generative AI strategies and adopt their core workflows, planning each phase of the process is essential. While generative AI feels like an immediate fire to address, projects and capabilities will continue to evolve and require a comprehensive 5 year strategy.
The leaders and teams that execute a well-built first POC that’s anchored in a strategy for scaling to other use cases will create competitive advantage in this AI automation race across industries.
Citations
PWC, 2024: https://www.pwc.com/us/en/library/ceo-survey.html