How To Structure A Conversation Design A/B Test
Before you standup your A/B test, there are a few things to consider before you even begin researching how your experience is performing. The first thing you’ll want to consider is which statistical approach you want to adopt to successfully run your A/B test and draw the right conclusion.
Frequentist and Bayesian reasoning are two different approaches to analyzing statistical data and making decisions based on it.
Personally, I’ve always used the Bayesian approach to A/B testing because the Bayesian approach has been know to provide actionable results faster and focuses on reaching statistical significance.
The Bayesian approach tells you the probability of variation A having a lower conversion rate than variation B or the control. It does not have a defined time limit attached to it, nor does it require you to have an in-depth knowledge of statistics. (source)
With that in mind, we’ll continue on with the expectation that we’ll be using bayesian statistics to measure the impact and efficacy of the A/B tests being run with your live assistant.
Research
Take a look at the conversational transcripts, historical conversation data, and usage of your interaction model. Where are some of the places where you’re getting a lot of no-match scenarios, or which intents and entities are the most popular and which aren’t? How often does the Bot handle all of your conversation versus where does a human need to step in?
Looking for areas of improvement in your bot can help you form a hypothesis tied to a metric your bot should be improving.
Formulate Hypothesis
Choose one metric from your reporting you’d like to influence with your A/B test. By making a data backed decision that focuses on a specific part of your conversation design, you can ensure confidence in the results from your test and make the appropriate changes to your skill. Testing an aspect of your skill without thinking about the impact you want to drive can lead to inconclusive tests.
The metrics you can impact are:
- NLU accuracy
- Human Handover Percentage
- Containment Rate
- Average Resolution Time
For a more in depth look at the metrics you should be tracking in your conversation design team, check out this resource from the Conversation Design Institute.
Create Variation
Make the variation to your skill that you would like to test. Your variation should directly relate to your hypothesis and should work to impact the metric you’ve focused your test around.
💡 A/B Testing Pro-Tip: You should only change one part of your A/B test at a time to be 100% sure that the results of your A/B test came from the single change that you did so you can learn how each change impacts user behavior. If you change too many aspects of your skill to test, you can’t be sure which change drove the results and can make your learnings messy.
Analyze and Deploy Changes
This is where your statistical analyzation preferences come in. If we’re using Bayesian statistics to measure the impact of your test, you can use this is A/B test calculator to measure statistical significance. Not only will this calculator let you know if you have reached statistical significance, it will also help you understand if your assistant has enough volume to reach statistical significance in an appropriate amount of time.
How To Structure Your Voiceflow Conversation Designs For A/B Testing
When you’re creating an A/B test within Voiceflow, you can split up your Control variation and your test variation using the Random blocks in the part of the design where you’d like to test a new variation.
Using the random step exactly where you’d like to put your test can help you ensure that you’re not recreating every other aspect of your conversation design that will remain the same as users navigate your conversational experience.

In the example above you can see that we wanted to test different openings for the bot to drive more use of intents that regularly went unused because visitors chose to go down the general help path.
The hypothesis for this test is that this opener will contain the conversation better and lead to less human intervention in the conversations.
A/B Testing In Conversational AI
Designing for optimal user experience rarely happens the first time you push a conversational experience live. Testing, iterating, and optimizing your chatbots, IVRs, voice, and in-car assistants is a process that can help you create the best experience possible for your users and learn more about your target customer.
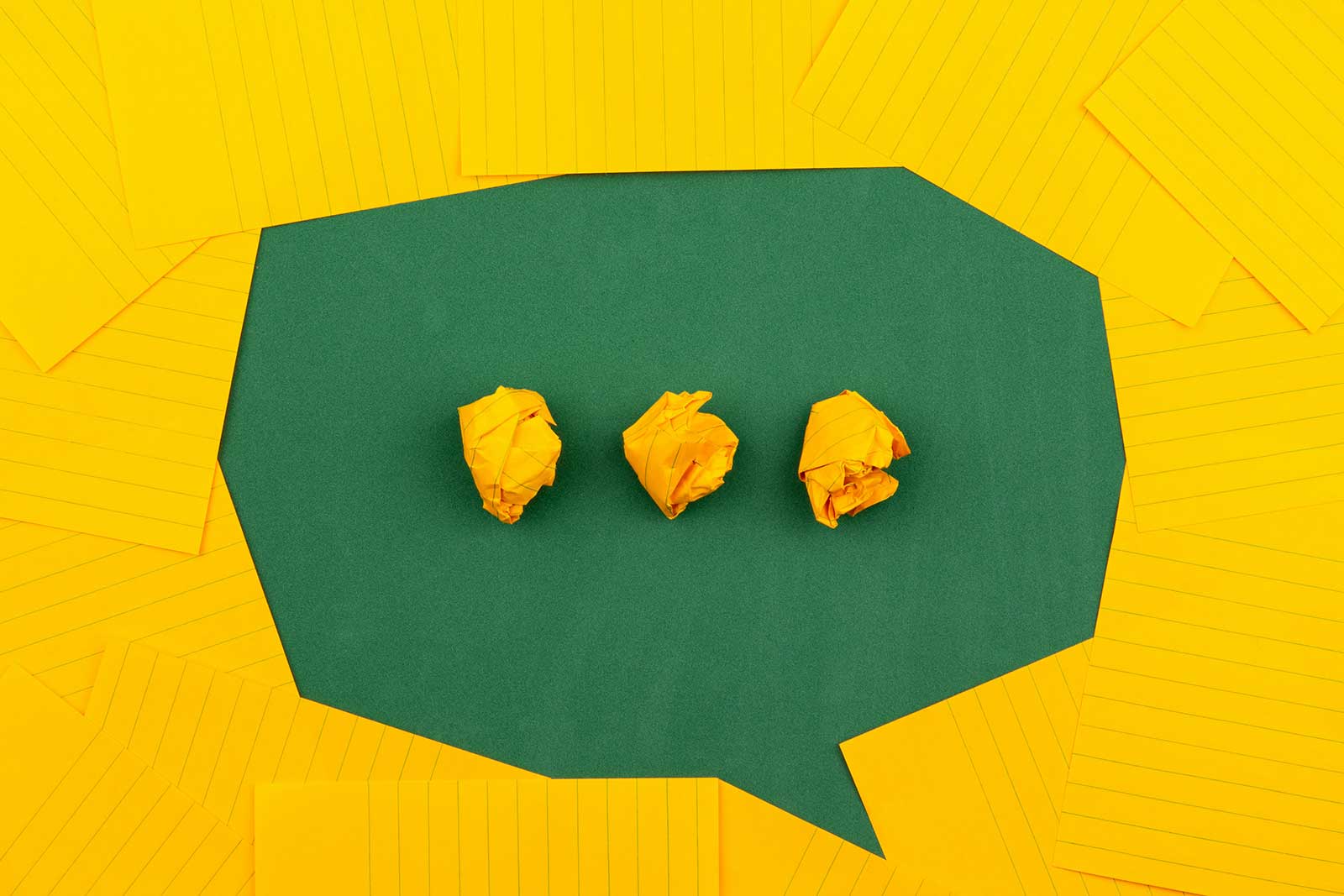